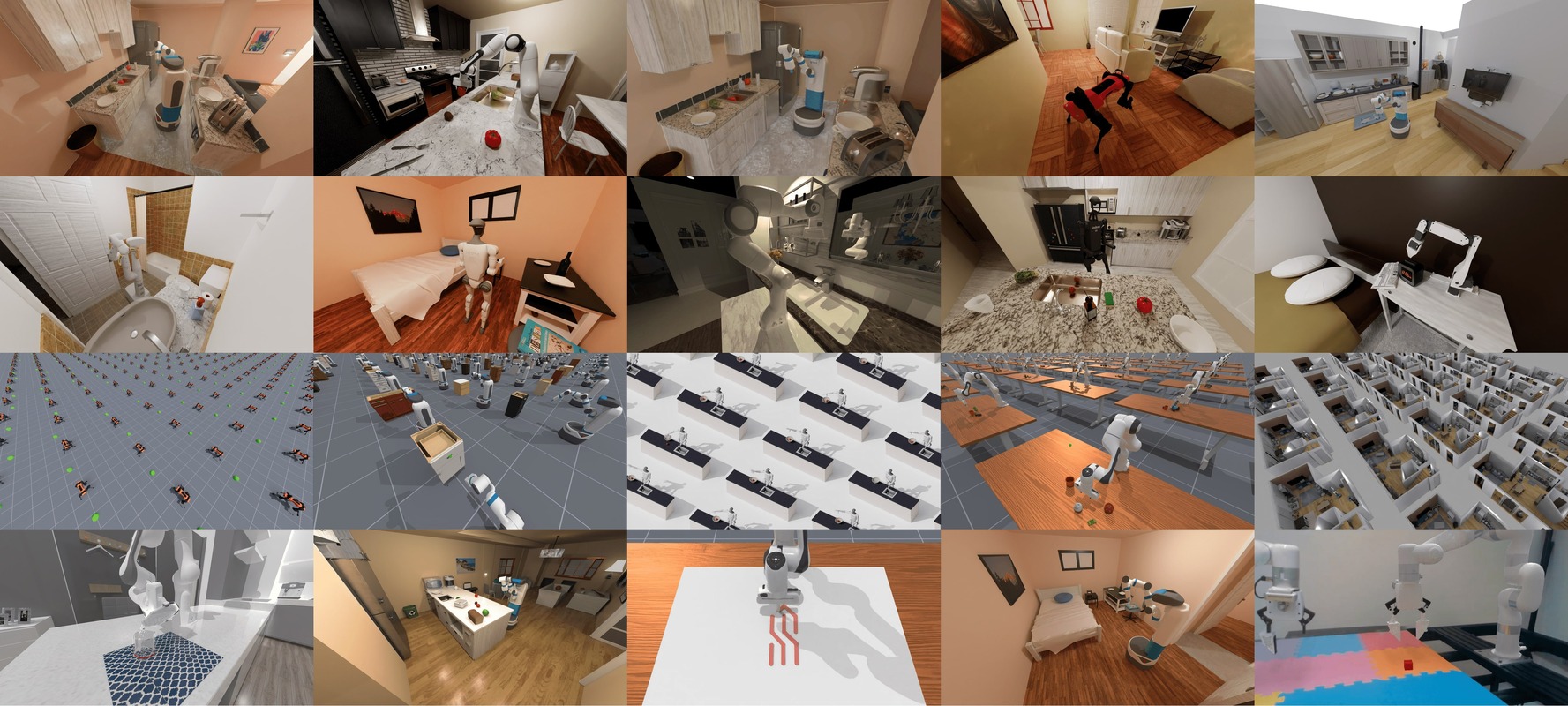
ManiSkill3: GPU Parallelized Robotics Simulation and Rendering for Generalizable Embodied AI
Stone Tao, Fanbo Xiang, Arth Shukla, Yuzhe Qin, Xander Hinrichsen, Xiaodi Yuan,
Chen Bao, Xinsong Lin, Yulin Liu, Tse-kai Chan, Yuan Gao, Xuanlin Li, Tongzhou Mu, Nan Xiao,
Arnav Gurha, Viswesh Nagaswamy Rajesh, Yong Woo Choi, Yen-Ru Chen, Zhiao Huang,
Roberto Calandra,
Rui Chen, Shan Luo, Hao Su
Robotics: Science and Systems (RSS) 2025
[project]
[paper]
[code]